The threat of cyberattacks looms larger than ever before. As cybercriminals develop more sophisticated methods, traditional security measures often fall short, necessitating innovative solutions. Enter artificial intelligence (AI), a game-changing technology that is rewriting the rules of cybersecurity management.
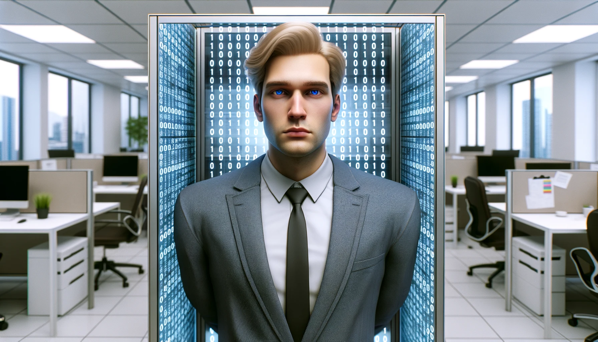
AI has positioned itself at the forefront of the cybersecurity landscape by enhancing capabilities such as threat detection and incident response. Techniques like user behavior analytics and anomaly detection not only identify potential breaches but also predict risks before they materialize. As organizations strive for more resilient security frameworks, AI serves as a catalyst for change, offering unprecedented analytical prowess and operational efficiency.
This article will explore six innovative ways AI is revolutionizing cybersecurity management, delving into its applications and benefits. From streamlining security operations to enhancing predictive maintenance, understanding these advancements is crucial for professionals aiming to bolster their organizations against evolving threats.
Overview of AI in Cybersecurity
Artificial Intelligence (AI) has become a critical asset in cybersecurity, significantly enhancing threat detection, vulnerability management, and incident response. By employing AI, organizations can boost their cyber resilience against sophisticated attacks. The use of AI and automation in cybersecurity not only reduces the average cost of data breaches but also speeds up the identification and containment of incidents.
AI applications in cybersecurity include real-time data analysis, automated threat detection, and behavioral pattern recognition. These capabilities enable the proactive identification of potential threats, allowing security teams to respond swiftly and effectively. Machine learning algorithms are pivotal in analyzing vast amounts of data, improving the accuracy and efficiency of threat detection over time.
The integration of AI into cybersecurity empowers the automation of response measures, enabling security teams to rapidly isolate threats based on predefined criteria. This automation is vital for addressing cyber threats, including phishing emails and malicious code, and managing security events. AI’s ability to analyze user behavior and network traffic in real time enhances the security posture by minimizing false positives and identifying anomalous behavior indicative of potential attacks, including zero-day attacks.
Advanced Threat Detection
AI significantly enhances advanced threat detection capabilities by employing machine learning algorithms to swiftly analyze vast amounts of data in real time. These technologies focus on identifying patterns and anomalies indicative of potential security threats. AI tools enable organizations to detect abnormal behavior and recognize zero-day attacks by scanning massive datasets quickly. Predictive analytics, powered by neural networks, consolidate data from multiple sources to highlight vulnerabilities and signs of ongoing attacks. This improves proactive threat detection. Furthermore, AI-driven automation streamlines incident response, allowing for faster and more efficient management of security incidents as they occur. Continuous learning capabilities ensure AI systems keep up with emerging threats, strengthening cybersecurity resilience overall.
User Behavior Analytics
User and entity behavior analytics (UEBA) systems leverage machine learning algorithms to scrutinize historical data, establishing behavioral norms for users and entities. This allows for the detection of abnormal activities that may indicate security threats. By monitoring real-time user activities, UEBA systems can spot deviations from established baselines, facilitating the early identification of potential account compromises. AI-driven user behavior analytics examine data such as login times and access patterns to highlight anomalies that suggest potential risks. The integration of AI in these systems supports proactive security measures by automatically blocking suspicious access or alerting security personnel. As AI systems continuously learn from new data, their detection capabilities improve, adapting to the evolving tactics used by cybercriminals.
Anomaly Detection Techniques
Anomaly detection involves identifying unusual patterns in data sources like logs and network traffic to alert on potential security threats. Machine learning algorithms excel in this area due to their ability to learn normal system behavior and identify deviations. Real-time monitoring and alerting are central to anomaly detection, with AI employing statistical methods to consistently analyze system activities for anomalies. This aids in discovering cyberattacks and operational issues by detecting outliers in system performance metrics. AI pattern recognition also assists in identifying user behavior issues, including accidental data leakage, by tracking and analyzing anomalies in user actions.
Enhancing Predictive Maintenance
AI has become a crucial component in cybersecurity, particularly in enhancing predictive maintenance. By analyzing vast amounts of network data in real-time, AI systems can identify patterns and anomalies that signal potential cyber threats. This proactive approach aids security teams in managing threats before they escalate, effectively boosting cyber resilience. Furthermore, AI-driven automation in incident response significantly cuts down response times, minimizing damage from cyber-attacks through efficient execution of predefined threat responses.
The implementation of AI leads to efficiency gains of 15% to 40%, allowing security operations to maintain or even improve their security posture with equivalent or fewer resources. Sophisticated AI technologies support the evolution of complex cybersecurity tasks such as improving threat detection and automating responses. By enhancing behavior-based security measures, AI can detect anomalous or suspicious behavior, offering early warnings of potential threats.
Incident Response Capabilities
AI revolutionizes incident response by automating reactions to frequent threats, which coordinates and executes rapid measures to mitigate security incidents effectively. By leveraging historical data, generative AI furnishes security analysts with strategies based on successful past tactics. This application streamlines the creation of incident response reports, enabling faster documentation and action.
AI’s ability to learn from past incidents allows it to continually refine and improve incident response strategies. By reducing response times and enhancing efficiency, AI-driven automation in incident response manages security threats more adeptly than traditional methods. This results in swifter and more effective management of security events, reducing the chances of damage from cyber threats.
Revolutionizing Network Microsegmentation
AI can dramatically improve the precision of microsegmentation in complex networks, enhancing overall security measures. By integrating AI and machine learning into microsegmentation tools, organizations can receive automated, identity-based recommendations for user access roles. This approach ensures appropriate data access levels and minimizes the risk of unauthorized data exposure.
AI technologies contribute to a more refined user identification process by increasing the granularity of grouping within security frameworks. With attribute-based access control, AI systems set clear guidelines on which roles can access specific devices, fortifying data protection protocols. This AI-driven approach is crucial in managing vulnerabilities more effectively.
Effective Access Controls
Artificial Intelligence enhances Identity and Access Management (IAM) by leveraging behavioral analytics and biometrics to strengthen authentication processes. This prevents unauthorized access and ensures that user identification is more accurate. AI-generated attribute-based access control further refines user roles, allowing only authorized access to sensitive data.
AI-powered identity management tools provide automated recommendations that align with users’ access needs, safeguarding sensitive information. These tools support enhanced zero trust security policies by tracking identification changes over time, ensuring ongoing compliance and effectiveness in access control. Organizations benefit from tailored security measures as AI analyzes user behaviors and contexts, bolstering their security and compliance posture.
AI in Vulnerability Management
Artificial Intelligence (AI) plays a crucial role in optimizing vulnerability management by efficiently identifying and prioritizing vulnerabilities. Leveraging AI, organizations can analyze potential impacts and the likelihood of exploitation, ensuring a more proactive approach to security. This not only highlights critical vulnerabilities but also allows security teams to focus their efforts where they are most needed, significantly reducing risk without increasing workload.
AI-based patch management systems automate the identification and remediation of security vulnerabilities. By minimizing manual intervention, these systems expedite the patching process, allowing for quicker responses to threats. Research indicates that 47% of data breaches stem from unpatched vulnerabilities, emphasizing the importance of AI-driven solutions for maintaining a robust security posture.
Identifying and Prioritizing Risks
AI-powered tools, such as Comply AI for Risk, provide comprehensive insights into risks, enabling organizations to assess both the likelihood and potential impact of threats. This empowers them to prioritize treatments effectively. Machine learning advancements enhance the detection capabilities beyond human limitations, identifying cyber threat indicators rapidly and efficiently.
Predictive analytics through AI applications facilitate foresight into potential future attacks. By integrating asset inventory data with threat exposure assessments, AI improves the precision of risk prioritization, highlighting areas most susceptible to breaches. Automated AI systems generate detailed risk reports, enhancing accuracy and reliability, and allowing security operations to address potential threats promptly and effectively.
The Role of Threat Intelligence
Cyber Threat Intelligence (CTI) is essential for gathering and analyzing information about potential cyber threats. By understanding these threats, security teams can proactively prepare for attacks before they happen. The integration of AI and machine learning in CTI automates routine tasks, allowing security professionals to concentrate on decision-making. AI provides actionable insights by organizing and analyzing threat data, enhancing the ability to predict and mitigate cyber threats.
Real-time alerts enabled by AI are vital for monitoring systems and responding swiftly to cyber threats. AI enhances proactive cybersecurity management by issuing timely notifications of potential attacks. In addition, effective threat intelligence aids incident response teams by offering a deeper understanding of current threats, thereby improving mitigation strategies. The use of AI helps to prioritize alerts, minimizing the chance of missing critical incidents due to the abundance of false positives and low-priority alerts.
AI-Powered Threat Analysis
AI is highly effective at identifying potential threats through data pattern analysis and anomaly detection. This capability allows organizations to anticipate and mitigate threats before they fully develop. Predictive analytics driven by AI offer early warnings, enabling the implementation of preventive strategies to avert breaches. Moreover, AI-driven automation optimizes incident response by swiftly identifying and isolating threats, which drastically reduces response times.
AI also enhances user behavior analytics by examining network behavior continuously. This helps in identifying deviations from normal patterns that could signify potential security threats. AI-powered security services like AWS GuardDuty utilize various data sources to detect abnormal behavior. They excel at recognizing unauthorized access attempts and detecting unusual network traffic spikes, reinforcing an organization’s security posture against sophisticated attacks.
Automated Security Operations
AI-powered automated threat detection solutions offer vast capabilities in processing immense volumes of network requests and endpoint activities in real-time. This technology significantly minimizes response time by rapidly identifying and addressing cyber threats, reducing the typical incident response timeline by an impressive 14 weeks compared to manual methods. By analyzing network traffic and user behavior, AI can distinguish between routine activities and potential threats, enhancing the security posture of organizations against sophisticated attacks.
AI also streamlines vulnerability management by pinpointing potential entry points for bad actors. It recommends necessary security updates, thereby reducing vulnerability exposure and fortifying defenses against zero-day attacks. This automation not only boosts security tool efficiency but also enhances the operational workflow of security teams, ensuring a swift and coordinated response against any cyber threat.
Streamlining Security Processes
AI technologies like Machine Learning and Predictive Analytics revolutionize the efficiency and accuracy of vulnerability management. By allowing security teams to focus on critical vulnerabilities, AI ensures that the highest-risk threats are addressed promptly. This reduces the time to detect and respond to cyber attacks, streamlining security operations and freeing up valuable resources for tackling more complex issues.
Generative AI plays a pivotal role in automating repetitive tasks in security operations, allowing analysts to concentrate on complex threats. By integrating data across various control points and employing entity behavior analytics, AI provides broader visibility, identifying threats faster than traditional methods. AI applications in cybersecurity yield efficiency gains between 15% and 40%, enabling organizations to achieve more effective security outcomes with the same or fewer resources.
Benefits of AI in Cybersecurity
Artificial intelligence (AI) plays a pivotal role in transforming cybersecurity by enabling organizations to move from reactive to proactive threat detection. AI systems analyze data in real time, identifying and preventing potential threats before they occur. These systems also enhance rapid response to security breaches, implementing automated measures that significantly minimize the impact and downtime associated with such incidents. Furthermore, AI continuously learns and adapts, which improves the accuracy of threat detection and reduces false positives, leading to enhanced overall security measures.
Cost Reduction
AI-driven automation in cybersecurity operations leads to significant cost reductions. By automating routine tasks such as log analysis and vulnerability assessments, AI minimizes the need for manual intervention. Additionally, by improving threat detection accuracy, AI reduces false positives, thereby preventing wasted resources on non-existent incidents. Organizations employing security AI and automation save an average of $1.76 million on data breach costs compared to those not utilizing these technologies, highlighting the financial benefits of AI integration.
Scalability and Flexibility
AI excels at analyzing vast amounts of data in real-time, allowing organizations to identify patterns and anomalies indicative of possible threats. This capability enhances the scalability of threat detection operations without additional resources. AI also enables automation in incident response, reducing response times and allowing security teams to efficiently manage numerous threats. Moreover, AI-powered solutions are adaptable to changing network conditions, dynamically re-evaluating security policies and access controls for continued strong defense.
Improved Accuracy and Speed
AI systems enhance threat detection and response efficiency by analyzing extensive data sets in real time. Machine learning algorithms enable AI to rapidly detect unusual behavior, including zero-day threats. Through generative AI, organizations can quickly identify new threat vectors by identifying patterns and anomalies. This technology streamlines security processes, quickening incident response and reducing response times. Generative AI also automates scanning of code and network traffic, providing detailed insights for better understanding and managing of cyber threats.
Challenges in Implementing AI
Implementing AI in cybersecurity brings significant challenges, especially for organizations with small or outdated datasets. These companies often find that AI underperforms, making traditional rule-based systems more effective for certain tasks. Additionally, a lack of necessary skills or resources can lead to errors in AI adoption, further complicating the process.
Transitioning to AI-based cybersecurity solutions is often complex and costly, especially for organizations reliant on legacy infrastructure. Inadequate hardware or cloud resources can also render AI deployment impractical. Furthermore, as AI is rapidly adopted, new vulnerabilities may emerge, requiring robust security protocols and regular updates to prevent exploitation by adversaries.
Technical Limitations
AI systems in cybersecurity come with technical limitations, such as producing false positives or false negatives. These inaccuracies can lead to inefficient resource use and potential security vulnerabilities. The complexity and lack of interpretability of AI models can also complicate troubleshooting and undermine trust in automated decision-making.
Significant computational resources are often required to implement and maintain AI systems, posing a cost barrier for many organizations. The integration of AI into existing security frameworks may also require substantial adjustments, complicating the process. Detailed documentation is crucial to mitigate issues and enhance understanding of these complex systems.
Workforce Adaptation
Incorporating AI into cybersecurity operations is shifting the focus of hiring practices. CISOs are increasingly prioritizing roles such as AI operators and fine tuners, who use prompt engineering skills to optimize security operations. This shift is facilitating the automation of repetitive tasks, allowing cybersecurity professionals to engage in more strategic work and boosting employee retention.
More than half of executives believe that AI tools will significantly improve resource and talent allocation within their cybersecurity teams. The adoption of AI and machine learning is already under consideration by 93% of IT executives, highlighting the growing reliance on these technologies to strengthen security capabilities and improve performance.
Real-World Examples of AI in Action
CrowdStrike
CrowdStrike employs AI technology to analyze and identify malware behavior in real-time. This proactive approach allows the system to effectively block malicious software before it can compromise systems or encrypt files. By preventing malware infections, CrowdStrike helps mitigate ransomware attacks, safeguarding critical infrastructures.
Case Studies from Major Enterprises
Many major enterprises have successfully integrated AI into their cybersecurity strategies to bolster their defenses against cyber threats. For instance, Wells Fargo employs AI-powered threat detection and response platforms that use advanced machine learning algorithms to analyze vast amounts of data in real-time, spotting patterns indicative of potential malicious activities. This capability significantly enhances their incident response times, as the system autonomously generates informed responses based on thorough data mining of security threats.
Amazon Web Services (AWS) exemplifies AI’s role in continuous security management through tools like AWS Inspector and AWS Macie. AWS Inspector continuously monitors and identifies security vulnerabilities within an organization’s AWS infrastructure, demonstrating the integration of AI for comprehensive security management. AWS Macie utilizes machine learning to discover and classify sensitive data, effectively protecting critical information such as personally identifiable information (PII) within cloud environments.
These case studies underscore AI’s crucial role in optimizing security operations. By improving threat detection and allowing security teams to focus on strategic priorities, AI helps organizations maintain a robust security posture in the face of increasingly sophisticated attacks.
More Information from MicroSolved
For more information on implementing AI-driven cybersecurity measures, MicroSolved is a valuable resource. They can provide insights into how AI enhances threat detection through real-time data analysis, leveraging behavioral recognition to identify both known and emerging threats. This approach moves beyond traditional signature-based methods, allowing for quicker and more accurate threat identification.
Organizations that incorporate AI into their security operations benefit from efficiency gains of 15% to 40%, enabling security teams to maintain or improve their performance with the same or fewer resources. Additionally, by using AI for predictive analytics and simulating attack scenarios, potential vulnerabilities can be uncovered, reducing the overall risk and cost of data breaches. This demonstrates the significant financial advantages of integrating AI in cybersecurity strategies.
MicroSolved can be reached for further assistance by email at info@microsolved.com or by phone at +1.614.351.1237. They offer guidance on protecting organizations against the increasing complexity of cyber threats through AI-enabled tools and practices.
* AI tools were used as a research assistant for this content.